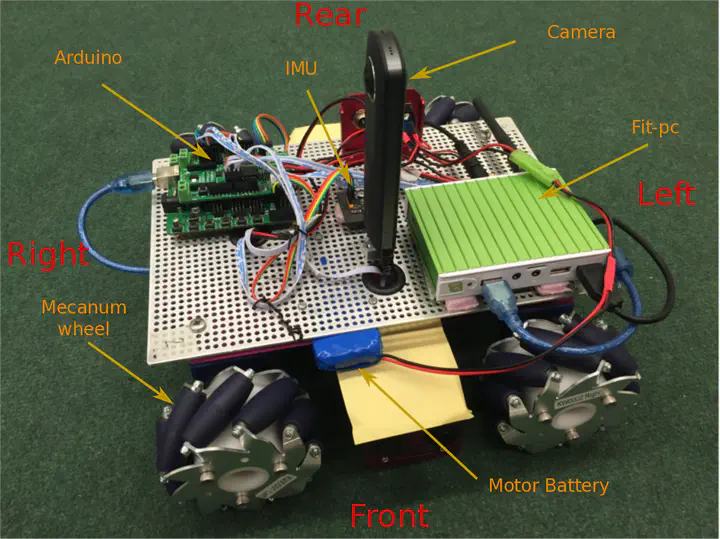
Analysing the behaviour of animals and studying their brain structure is a common way to create bio-inspired artificial intelligence methods. Fiddler crabs are animals with specific limitations on their sensors that have a great ability of evading on the presence of potential predators. In this project we study these animals with respect to the physiology of their unique sensor – their vision – and their evading manoeuvres, in order to create a machine learning model, which imitates this interesting behaviour. More specifically, we propose a semi-supervised architecture of neural network, inspired by the structure of fiddler crabs’ brain and their evasion behaviour’s feedback loops. We combine location specific visual units and LSTM recurrent units in order to build a model capable to adapt this behaviour. We trained our model using a data-set we created using data from experiments done with living crabs in their habitat. Comparing our statistical results of our model’s behaviour with the ones of the original crabs behaviour we show that this model captured some key features of this behaviour as well as it seems to behave quite realistic in the simulations. Therefore, we show that it is reasonable to consider machine learning models as potential solutions in animal behaviour adaptation problems.