Insect neuroethology of reinforcement learning
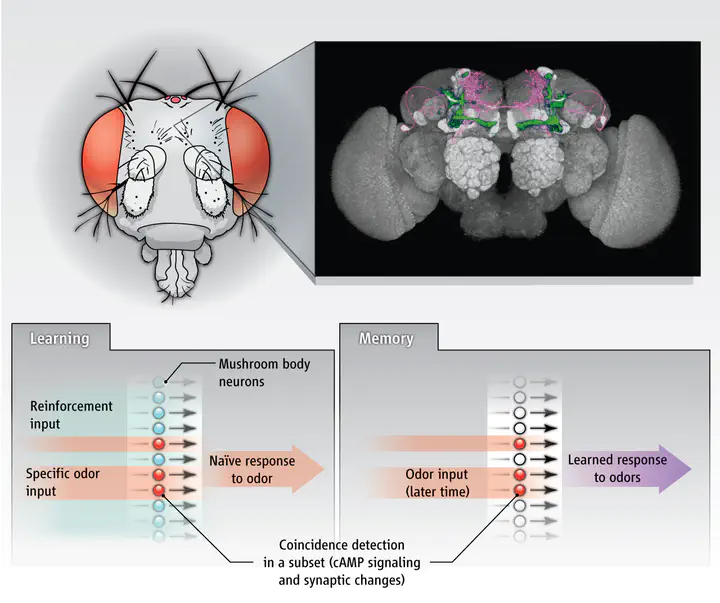
Historically, reinforcement learning is a branch of machine learning founded on observations of how animals learn. This involved collaboration between the fields of biology and artificial intelligence that was beneficial to both fields, creating smarter artificial agents and improving the understanding of how biological systems function. The evolution of reinforcement learning during the past few years was rapid but substantially diverged from providing insights into how biological systems work, opening a gap between reinforcement learning and biology. In an attempt to close this gap, this thesis studied the insect neuroethology of reinforcement learning, that is, the neural circuits that underlie reinforcement-learning-related behaviours in insects. The goal was to extract a biologically plausible plasticity function from insect-neuronal data, use this to explain biological findings and compare it to more standard reinforcement learning models. Consequently, a novel dopaminergic plasticity rule was developed to approximate the function of dopamine as the plasticity mechanism between neurons in the insect brain. This allowed a range of observed learning phenomena to happen in parallel, like memory depression, potentiation, recovery, and saturation. In addition, by using anatomical data of connections between neurons in the mushroom body neuropils of the insect brain, the neural incentive circuit of dopaminergic and output neurons was also explored. This, together with the dopaminergic plasticity rule, allowed for dynamic collaboration amongst parallel memory functions, such as acquisition, transfer, and forgetting. When tested on olfactory conditioning paradigms, the model reproduced the observed changes in the activity of the identified neurons in fruit flies. It also replicated the observed behaviour of the animals and it allowed for flexible behavioural control. Inspired by the visual navigation system of desert ants, the model was further challenged in the visual place recognition task. Although a relatively simple encoding of the olfactory information was sufficient to explain odour learning, a more sophisticated encoding of the visual input was required to increase the separability among the visual inputs and enable visual place recognition. Signal whitening and sparse combinatorial encoding were sufficient to boost the performance of the system in this task. The incentive circuit enabled the encoding of increasing familiarity along a known route, which dropped proportionally to the distance of the animal from that route. Finally, the proposed model was challenged in delayed reinforcement tasks, suggesting that it might take the role of an adaptive critic in the context of reinforcement learning.