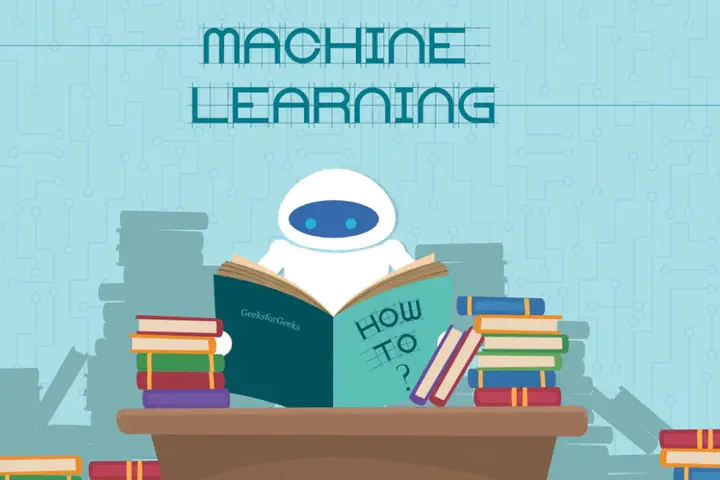
Many scientists, who specialize in supervised learning, are interested in multi-label data for two main reasons. First of all, this type of data arising at a variety of applications such as semantic indexing of documents, music and video, protein function prediction, medical diagnosis, drug discovery and search engine queries clustering. Secondly, multi-label data perform interesting research challenges like the use of correlations between labels and scaling to a large number of labels.
In multi-label data, every subject of interest is characterized by one or more labels from a label set and the purpose is not a simple classification of an instance, but a label ranking for their relevance to the subject or a bipartition of them to these that are relevant and those which are not (multi-label classification). In this project, we investigate this field and we apply a deep learning method, inspired by the theory that explains how the brain recognizes patterns. Technology companies are reporting startling gains in fields as diverse as computer vision, speech recognition and the identification of promising new molecules for designing drugs.
We present a fairly recent kind of neural network, the deep belief network, which handles multi-label data without transforming them before or after the training. This transformation is undesirable, because it causes the dataset to be bigger. Our aim is to build a model that can handle multi-label data using deep learning techniques. In this project, we showed that these techniques – specifically the deep learning using belief networks – have better results in the most of the encountered subjects of interest